The global engine of financial markets ventures into the cloud
Nasdaq manages 30 stock exchanges across North America and the Nordics, and is the home of over 4,000 public companies. They also provide technology to 2,200 financial institutions in 130 markets. To say Nasdaq is the engine of the financial markets is not a hyperbole.
In 2012, before most of the financial community made the jump, Nasdaq started their journey to the cloud. They quickly progressed—launching a data warehouse, new products, and new marketplaces. After encountering scalability issues, they migrated to a data lake and optimized their data infrastructure by 6x.
The migration saved significant maintenance costs and opened the door for analytics, ETL, reporting, and data visualization use cases.
An opportunity arises from a better data infrastructure: analytics
Opportunity 1: Empowering business users
Business users at Nasdaq previously struggled to access the data they needed. Data requests were a complicated process, requiring business stakeholders to submit tickets in order to receive the reports they needed. The reports could be provided by any of Nasdaq’s four distinct data teams—who owned which data product was unclear, which created confusion and overlap.
“Our vision was to have business users participate in the transformation of our data logic,” said Michael Weiss, Senior Product Manager at Nasdaq.
Opportunity 2: Provide new types of data access to clients
As the cloud-native data stack developed, so did the requirements of Nasdaq’s business clients. Delivering static reports was no longer enough. With their unique datasets in hand, Nasdaq saw an opportunity to build data products for these new needs.
“Clients were becoming increasingly more likely to ask for dynamic access data, whether it be APIs or visuals,” said Michael. “We then searched for tools that could solve this new demand.”
But to get there, Nasdaq still needed to overcome several roadblocks.
Data for analytics: not as simple as it sounds
Data optimized for performance, not for querying
Nasdaq’s unique trading data is both a valuable differentiator and their biggest challenge for analytics use cases.
“Trading system data is really complex. It is optimized for performance, not for querying or analytics as most message data is,” said Michael.
The large data volume also added an extra layer of complexity. In 2019, Nasdaq operated 25 to 35 billion transactions daily through their “data warehouse-lake.” But, after 2020, it increased to 100-125 billion per day.
Legacy ETL tools locked business users out
One of the main blockers in onboarding business users to data modeling was Nasdaq’s legacy data tools. They used a combination of Pentaho and “a lot of SQL scripts running around for various things.”
“Even though business users wanted to engage with the data, our legacy data infrastructure didn’t allow it,” explained Michael.
Teams working in silos
Nasdaq’s teams therefore resorted to creating their own data stacks for their reports. Because new data requests took weeks or even months to address, teams would try to solve their own data requirements.
“Business users couldn’t sit around and wait for the reporting team to add a column to a report, get insights into making a pricing change, or see what's actually happening in their markets,” said Michael.
Monolithic SQLs script
SQL scripts were an important part of Nasdaq’s data infrastructure. Different data teams were using SQL for analysis data ingestion. However, a lack of documentation and collaboration meant they were becoming monoliths.
“SQL scripts were hard to maintain. When the person in charge of them would leave the company, we’d have no idea what was in there. And then we had to rewrite the code,” said Michael.
Moving to a secure, modern data stack with the support of dbt Cloud
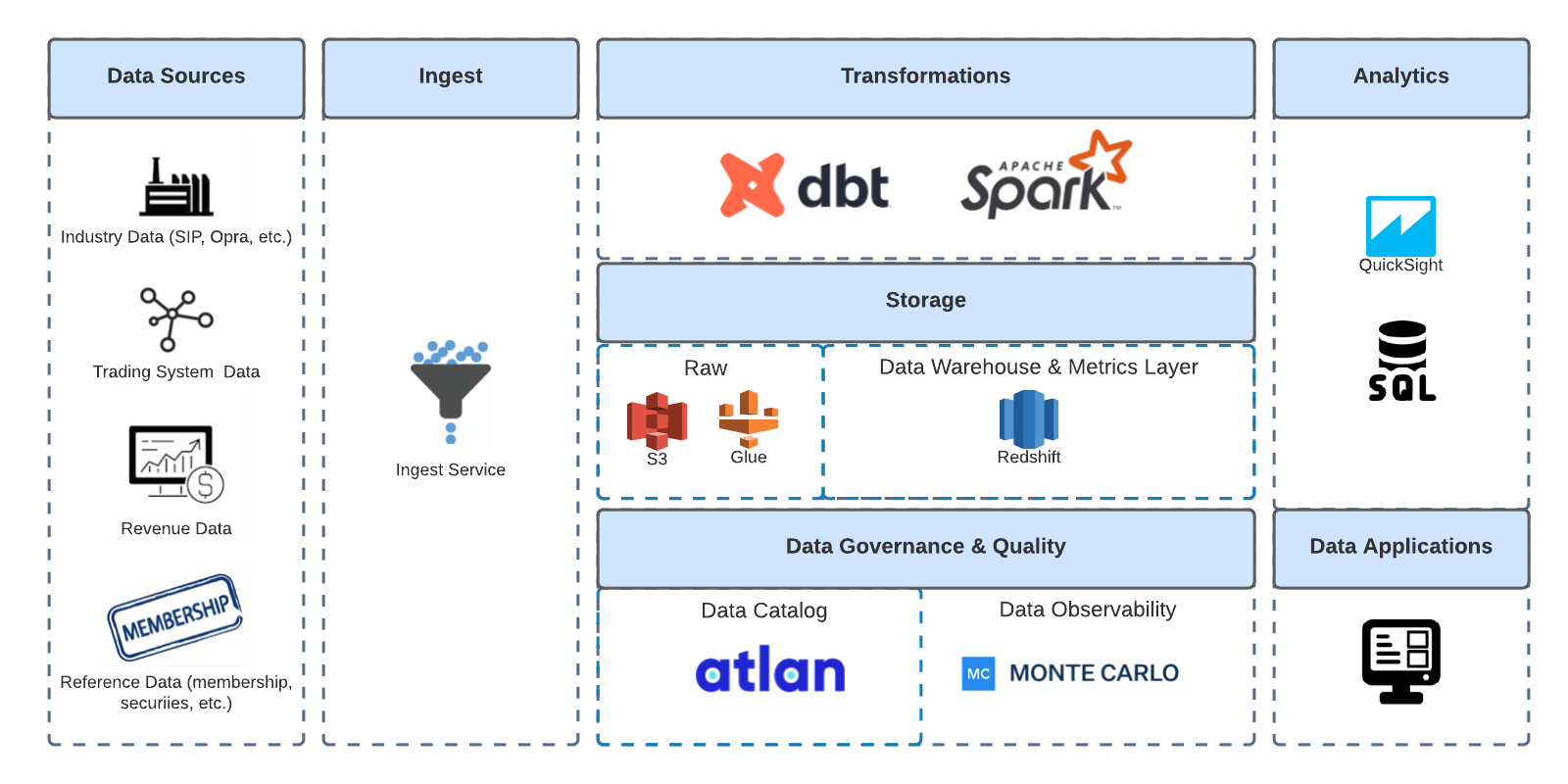
Building for end users: sales and executives
It was clear from the get-go that a move to a different data stack wasn’t just about the tooling, but about the end user. Nasdaq’s team had a vision to onboard business users into the data modeling process—their new tools needed to enable team members across the company with different technical skills to participate.
“It’s about the people you pick tools for,” explained Michael. “And it’s hard to pick tools for sales people and executives.”
Success meant unlocking a previously-unattainable velocity for Nasdaq’s business teams. They would no longer have to wait for reports and miss the window where an insight could change the outcome of a business decision.
“To me, self-service is not just necessarily about the capability of someone to get access to data, but how quickly you can give them the answer to something that they don't have the answer to today,” shared Michael.
dbt’s Enterprise support sets the new data stack up for success
One of Nasdaq’s concerns about moving to a new stack was onboarding analysts. Setting up development environments was something most analysts had never done before.
“I didn't want to have to sit with each team member and guide them through a complex install as we brought in new analysts,” said Michael.
dbt Labs’ services team stepped in, helping Nasdaq’s large teams onboard with ease and move into a productive implementation in a matter of weeks. The team at dbt Labs continued to assist with Nasdaq’s large-scale dbt roll-out to answer more challenging setup questions and optimize their models at scale.
“dbt Labs laid out good practices and helped really get our options business, which was the first business we got online, moving quickly,” said Michael.
“Most recently, we had performance issues with a model that does 15 to 20 billion messages per day. The dbt Labs services team helped us solve that. The model took 45 minutes to an hour to run in a day, but they got it down to 10 minutes.”
Streamlined analytics for both data and business teams
Better collaboration with SQL
Following the set-up of dbt, SQL models provided new value for Nasdaq. The models were now understandable, maintainable, and repurposable.
“I was very skeptical of improving collaboration through SQL, but it actually worked,” laughed Michael. The combination of SQL’s accessibility and dbt’s embedded software engineering best practices simplified and centralized Nasdaq’s analytics workflow across teams.
Insights from previously inaccessible data points
Nasdaq’s most competitive business, their options business, was the first to fully onboard to dbt Cloud. They can now count on several hundred models that lead into a couple key models they use day-to-day.
“Because of these dbt models, our sales and executive teams, can now see data points they never had access to before,” said Michael. “For example, exchanges do pricing changes on a relatively monthly basis in the US. It has an impact on all types of different metrics you care about: your revenue, your volume, who's trading with who, things like that.”
No more missed business opportunities from slow turnaround times
The move to a modern data stack completely changed how business teams access data at Nasdaq.
“Before, our sales and executive teams would put a request into the economic research team or the data team. If they were lucky, it would be in a report somewhere or several reports, and maybe they could piece that answer together. Nine times out of 10, that was not true,” shared Michael.
The wait time for these reports could take months.
“By the time business users would receive what they requested, it might not even be relevant anymore. They could have missed a new business opportunity or lost revenue. Data velocity is important,” explained Michael.
“In today's world, because we have built a robust set of models and metrics, people can model or view the data themselves.”
What’s next: Building on top of the modern data stack
From the start, Nasdaq’s approach to building a modern data stack was agile. They built piece by piece to isolate successes, mitigate risk, and iterate without a complete infrastructure overhaul every time.
Onboarding new teams and markets
The options business is fully online and reaping the benefits, but there are still other businesses to go. For example, the equities team is next in line to implement dbt.
Currently, Nasdaq is onboarding their economic research team to dbt. They have their own backlog of scripts and models ready to be exposed and repurposed as dbt models.
Nasdaq is also looking at how they can extend their new data stack to international markets, like the Nordics.
Adding new tooling and capabilities
Nasdaq signed new contracts with Atlan and Monte Carlo, and are now working on integrating the tools. Atlan will unlock an improved view of their data lineage, and Monte Carlo will enable a more proactive approach to data pipeline breaks.
Another tool—a reverse ETL—is potentially on the horizon:
“Our work on this is never done. We're always going to constantly evaluate and make sure we're picking the right tools for the right job,” emphasized Michael.